AI chatbots have revolutionized customer service in recent years, offering 24/7 engagement capabilities and human-like conversation. A recent study by Gartner predicts that by 2025, 80% of customer service and support organizations will be applying Generative AI to improve their customer experience and enhance agent productivity. However the quick-paced, widespread adoption of such technologies comes with its fair share of risks. A few recent notable incidents reveal the significant risks associated with AI chatbot miscommunications and their impact on brand integrity.
Generative AI will inevitably continue to impact the customer experience landscape in the coming years. Therefore it’s more important than ever to understand the associated risks and implement strategies to mitigate them, ensuring they don't escalate into public controversies.
The Risks of Miscommunication
The seamless integration of chatbots into our daily operations offers the promise of increased efficiency and personalized customer service. Yet as we navigate this new frontier, the importance of AI testing and certification is plain as ever in recent news. Take for instance the recent Air Canada chatbot incident, where a simple miscommunication spiraled into widespread frustration over a customer’s incorrect bereavement refund policy information; this cast a spotlight on the delicate balance of trust between consumers and brands. Similarly, a GM dealership witnessed firsthand the financial jeopardy (and humorous blunder) of AI missteps when a chatbot offered a Chevy Tahoe at the absurd price of $1. While this seems like a great deal for the consumer, it underlines the unforeseen pitfalls in these automated chatbot systems. Another, more vulgar example, is when a parcel delivery firm known as DPD had to disable their chatbot system after it was coaxed into swearing at a customer.
These incidents are not anomalies – they’re cautionary tales. They stitch together a common thread underscoring the crucial role of AI testing and certification in this new chatbot-heavy customer support world.
A PwC report notes that 42% of consumers would stop using a brand after a single negative experience – underscoring the high stakes of these AI interaction failures.
Companies should focus on learning from these cautionary tales by prioritizing stringent internal AI governance. By learning from these examples we can steer a course towards a future where AI promises both innovation and reliability.
Why Do Chatbots Misstep?
Chatbots powered by Large Language Models (LLMs) are becoming increasingly integral to customer service ecosystems. However, advancement brings to light two pivotal challenges: overgeneralization and pleasing bias. These two issues highlight the balance required between delivering accurate information, and achieving high levels of user satisfaction.
Overgeneralization occurs when chatbots struggle to provide information that accurately reflects a brand’s specific policies or offerings (despite being trained on the company’s vast datasets). This stems from the limitations of said datasets used for training these models – while expansive, they may not encompass the latest or most niche details about a company’s products or services. This can lead to responses that are overly generic or occasionally incorrect. The lack of specificity can frustrate users seeking precise information, undermining both the chatbot’s utility and the brand credibility.
Pleasing bias compounds this challenge. Pleasing bias is the tendency for chatbots to make promises that may not align with reality. As chatbots are designed to engage users in a helpful and supportive manner, they aim to fulfill user requests to the best of their ability. However this inclination can backfire – as humorously shown by the Chevy Tahoe incident. Pleasing bias stems from chatbots’ programming and training objectives, which prioritize the user’s satisfaction. In the effort to generate agreeable responses, these digital assistants may inadvertently generate false information.
This bias isn’t just a byproduct of chatbot design. It’s deeply ingrained in the training data, which includes human conversation, interaction, and strategies of politeness and affirmation. Not to mention, if a chatbot’s reinforcement learning is also calibrated to optimize user satisfaction metrics, it could learn that pleasing the user yields “better performance”. Minimizing negative feedback and maintaining user engagement can lead chatbots to navigate conversations that avoid upsetting the user – sometimes at the expense of accuracy. The current state of AI technology, while advanced, still falls short of comprehensively grasping and responding to the full spectrum of human subtleties. This leads chatbots to default towards overly accommodating or optimistic responses.
Addressing pleasing bias requires a multifaceted approach. Data scientists could recalibrate the training data to balance positive and negative outcomes better, and adjust the model’s objectives to emphasize accuracy over pleasing the user. They can also introduce fallback mechanisms that will escalate incoming complex queries to human operators to mitigate the risk of misinformation. Fostering transparency and carefully managing the users’ expectations can also help. By refining these chatbot responses and setting reasonable standards, developers can navigate the line between maintaining engagement and ensuring reliable information is being given to customers.
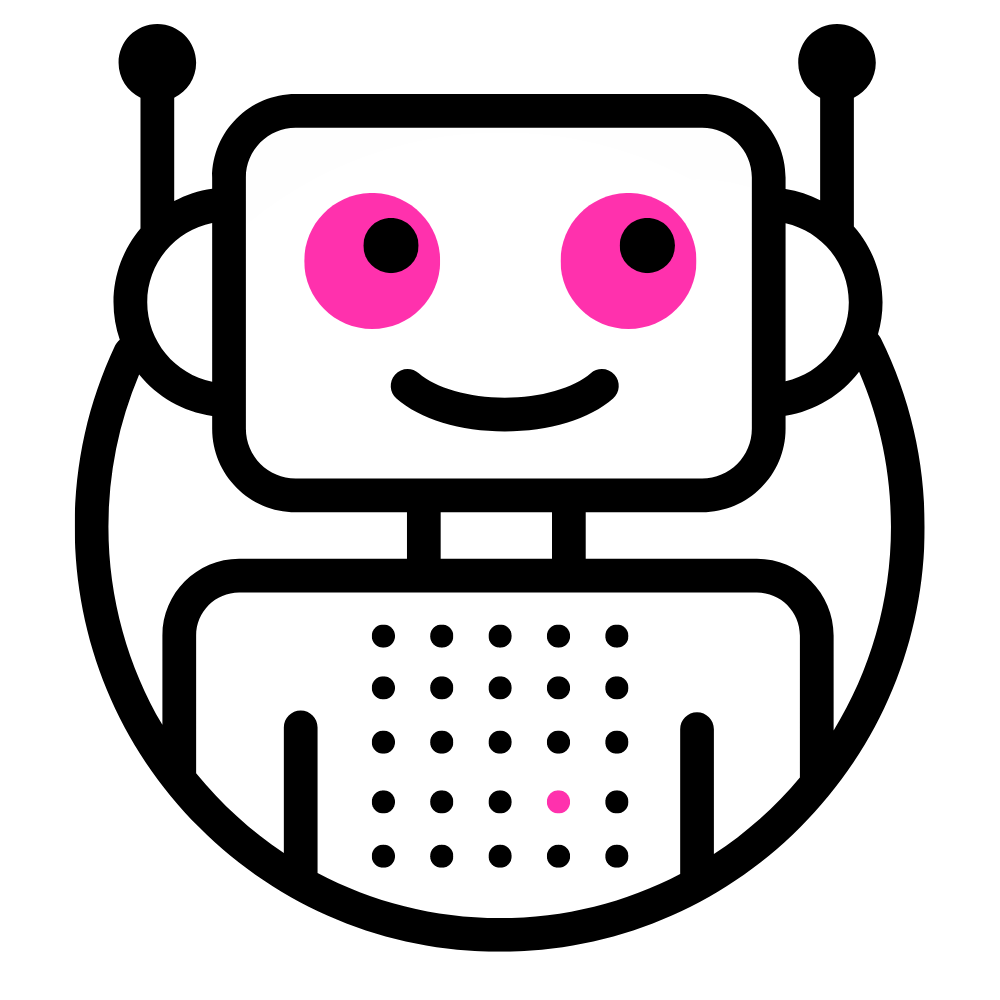
Need help answering the following questions?
Talk it over with our AI bot Xybo.
How AI Testing & Certification Protects Your Brand
Navigating this new frontier of AI integration into customer services brings both opportunities and unforeseen challenges. The implementation of AI chatbots carries inherent risks that if not properly managed, can detract from the customer experience. This is where Xyonix steps in with a thoughtful approach to AI testing and certification.
Precision Testing and Certification: At Xyonix, we employ a comprehensive assessment methodology designed to align chatbot performance with your brand’s values and accuracy requirements. Our process involves rigorous benchmarking against industry standards for AI performance, such as accuracy rates above 95% in understanding and responding to user queries. This precision testing ensures that your AI chatbots reflect the reliability your brand stands for.
Adaptive Learning and Updating: Our approach incorporates continuous learning models, as the nature of brand policies and customer expectations are ever-evolving. Our models are designed to keep your AI chatbots ahead of the latest developments in your company’s offerings and policies. Our adaptive learning systems ensure that your chatbot evolves in real-time, maintaining its effectiveness in every interaction.
A study from McKinsey indicates that continuous learning models can half the error rates of AI systems. Our strategy isn’t just about risk mitigation – it’s about setting a new benchmark for AI performance that prioritizes precision and adaptability.
OUR Commitment to Responsible AI
Integrating AI chatbots into your customer service arsenal is a promising way to boost efficiency and customer loyalty – but also demands vigilance. Learning from public past missteps is crucial. With Xyonix as your partner, you’re not just addressing potential pitfalls of AI miscommunication; you're embracing a future where AI technologies are more reliable and bolster brand integrity.
AI project success is crucial, but challenging, with 47% of organizations reporting at least one AI project failure as noted by IDC - highlighting how paramount the importance of thorough testing and certification is.
Xyonix stands ready to ensure your AI initiatives not only meet the mark, but significantly elevate your brand’s reputation in the eyes of your customers. Explore how Xyonix’s premier testing and certification services can transform your AI chatbot strategy, forging a path to unparalleled customer engagement and brand loyalty.
SIGN UP FOR YOUR FREE AI RISK ASSESSMENT
Sources:
BBC News. (2022, July 21). Title of the article. Retrieved from https://www.bbc.com/news/technology-68025677
McKinsey & Company. AI-Driven Operations: Forecasting in Data-Light Environments. Retrieved from https://www.mckinsey.com/capabilities/operations/our-insights/ai-driven-operations-forecasting-in-data-light-environments
Gartner. (2023, August 30). Gartner Reveals Three Technologies That Will Transform Customer Service and Support by 2028. Retrieved from https://www.gartner.com/en/newsroom/press-releases/2023-08-30-gartner-reveals-three-technologies-that-will-transform-customer-service-and-support-by-2028
Tech.co. List of AI Failures: Mistakes and Errors. Retrieved from https://tech.co/news/list-ai-failures-mistakes-errors
Lexalytics. (2020). Stories of AI Failure — and How to Avoid AI Fails. Retrieved from https://www.lexalytics.com/blog/stories-ai-failure-avoid-ai-fails-2020/
PricewaterhouseCoopers. PwC’s Global Artificial Intelligence Study: Exploiting the AI Revolution. Retrieved from https://www.pwc.com/gx/en/issues/data-and-analytics/publications/artificial-intelligence-study.html